Having “grown up” in a world of small-n, non-parametric analyses of animal research, I often find myself having to spruce up my basic understanding of statistics. Since my graduate school days in the 70s, the computer explosion seems to have fueled our abilities to conduct more and more sophisticated analyses. I am finding it nearly impossible to maintain my expertise in psychology, while keeping up simultaneously with computer science and statistics. My solution is to collaborate with people who ARE expert in those areas, but I still need to maintain enough understanding to collaborate, to apply new methods to design, and to read results sections intelligently (which can be very intense when reading about fMRIs, etc.)
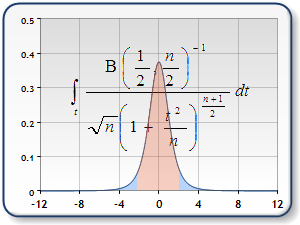
Formulas are grand, but I love those numerical examples
So I embark on a quest to update myself in statistics, but I find that not only have the statistics changed, but the methods of teaching them have as well. None of the textbooks or online resources seem to offer too many examples of how to do things. The “discovery” method, which suggests that if a student figures something out on his/her own, it sticks better, seems to be the order of the day. The problem with that approach is that it takes a lot of time, which I don’t have. When I expressed my frustration to a colleague in Math, he described learners like myself as “cooks.” Give us a recipe, and we’ll give you a consistent outcome every time. That philosophy may not produce the greatest mathematical thinkers of the age, but it does allow us to perform accurately and reliably in what we need to do.
Einstein, who wasn’t too shabby in math, said “example isn’t another way to teach. It’s the only way to teach.” I noticed as my daughters went through K-12 that their math books had few examples, and they did very few problems as homework. I can’t imagine how anyone learns to be comfortable with math that way. I learn best by seeing a formula applied to a numerical example several ways. My mind sort of reverse engineers that process, I can see why the numbers go where they go, and I understand the process. Unfortunately for me, my rather alarming stack of statistics textbooks are very cryptic. You’re lucky if you find any numerical examples at all! Online resources seem keen to provide syntax and java scripts for just about everything, but this is kind of a black-box approach for those of us who are trying to understand WHY procedures are being done.
So much to my relief, I revisited an old “friend,” Geoffrey Keppel, now retired and emeritus at Berkeley, by obtaining every textbook he ever wrote. It appears that Professor Keppel has continued to publish his remarkably clear statistics textbooks following the 1973 dog-eared, coffee-stained, highlighted copy that was my best friend in graduate school. And ta da! The books are replete with the clearest of explanations and best of all–numerical examples! I am not surprised that Professor Keppel received a Distinguished Teaching Award at Berkeley.
Statistical literacy is one of the cornerstones of a democracy. If we cannot evaluate published statistics intelligently, how can we make good decisions? Rebecca Goldin has a great article on how statistics are misused in the media. She lists the following as the most common mistakes:
- The difference between causation and correlation
- The meaning of statistically significant
- Orders of magnitude, and the “prevalence” of a problem
- Confounding factors
- Relative risk versus absolute risk
- Margin of error
- The importance of scientific consensus
Maybe if more statistics materials were like Keppel’s, we wouldn’t be making these errors so frequently.
1 Comment
ylabiaga · November 1, 2009 at 11:19 pm
I took an intro to statistics class my second year at Cal Poly, and it proved to be pretty useful. My teacher, Soma Roy, was very good and I learned that statistical literacy is crucial when interpreting studies, especially in the media because often they can be portrayed as biased. For example, causation is NOT the same as correlation. And statistical confidence depends on your margin of error. I believe everyone needs to know these basic definitions of statistics…what if you’re reading a study on a new drug and you don’t know what the difference between relative and absolute risk is? That could be deadly! I highly agree: Statistical literacy is very important.
Comments are closed.